P-gp Inhibitors: Difference between revisions
No edit summary |
|||
(6 intermediate revisions by 2 users not shown) | |||
Line 10: | Line 10: | ||
===Features=== | ===Features=== | ||
* The P-gp Inhibition prediction module uses two models to estimate the probability a compound inhibits P-gp and if so, is it a potent inhibitor? | * The P-gp Inhibition prediction module uses two models to estimate the probability a compound inhibits P-gp and if so, is it a potent inhibitor? | ||
* Additional knowledge-based | * Additional knowledge-based model classifies compounds as P-gp inhibitors/non-inhibitors on the basis of relevant structural features and basic physicochemical parameters. | ||
* Statistical algorithms calculate estimate the reliability of every prediction by means of Reliability Index calculation | * Statistical algorithms calculate estimate the reliability of every prediction by means of Reliability Index calculation. | ||
* Displays experimental data for the 5 most similar compounds from '''P-gp DB''' | * Displays experimental data for the 5 most similar compounds from '''P-gp DB'''. | ||
* Training set consisted of >1,500 compounds. Reference data were compiled from more than 800 original publications. | * Training set consisted of >1,500 compounds. Reference data were compiled from more than 800 original publications. | ||
<br /> | <br /> | ||
Line 48: | Line 48: | ||
<div class="mw-collapsible | <div class="mw-collapsible"> | ||
==Technical information== | ==Technical information== | ||
Line 66: | Line 66: | ||
===Reference database=== | ===Reference database=== | ||
ACD/Percepta includes a browsable P-gp DB comprised of 2,290 compounds with experimental data related to their interactions with P-gp. Each compound in the DB is classified as a P-gp substrate or non-substrate (inconclusive or contradictive data are marked as Yes/No or No/Yes in the ''''Substrate''' field) and efficiency of P-gp mediated transport is provided for substrates. '''High efficiency''' describes compounds that are transported with the rate similar to the best substrates (vinblastine, daunorubicin, paclitaxel). Similarly, drugs comprising the database are classified according to their '''Inhibitor''' liability. '''Potency''' field denotes effectivity of P-gp inhibition, '''High potency''' representing compounds that inhibit P-gp as good as standard inhibitor verapamil or even better. | |||
In the '''Assays''' section, the methods that were used in the analysis of P-gp substrate/inhibitor specificity are listed: | In the '''Assays''' section, the methods that were used in the analysis of P-gp substrate/inhibitor specificity are listed: | ||
Line 76: | Line 76: | ||
* '''P-gp ATPase modulation''' – activation or inhibition of P-gp ATPase. This assay does not differentiate P-gp substrates and inhibitors. | * '''P-gp ATPase modulation''' – activation or inhibition of P-gp ATPase. This assay does not differentiate P-gp substrates and inhibitors. | ||
===Model features & prediction accuracy=== | |||
The predictive models of P-gp inhibition were derived using GALAS (Global, Adjusted Locally According to Similarity) modeling methodology (please refer to [http://www.ncbi.nlm.nih.gov/pubmed/20373217] for more details). | |||
Each GALAS model consists of two parts: | |||
* Global baseline statistical model employing binomial PLS with multiple bootstrapping using a predefined set of fragmental descriptors, that reflects general trends in P-gp inhibitor specificity. | |||
* Global baseline statistical model employing binomial PLS with multiple bootstrapping using a predefined set of fragmental descriptors. | * Similarity-based routine that performs local correction of baseline predictions taking into account the differences between baseline and experimental values for the most similar training set compounds. | ||
* | <br> | ||
GALAS methodology also provides the basis for estimating reliability of predictions by the means of calculated Reliability Index (''RI'') value that takes into account: | |||
* Similarity of | * Similarity of tested compound to the training set molecules (prediction is unreliable if no similar compounds have been found). | ||
* | * Consistence of experimental values and baseline model prediction for the most similar similar compounds from the training set (discrepant data for similar molecules, i.e. alternating P-gp inhibitors and non-inhibitors lead to lower ''RI'' values). | ||
Reliability Index ranges from 0 to 1 (0 corresponds to a completely unreliable, and 1 - a highly reliable prediction) and serves as an indication whether a submitted compound falls within the Model Applicability Domain. Compounds obtaining predictions ''RI'' < 0.3 are considered outside of the Applicability Domain of the model. | |||
<br><br> | |||
The presented method also forms the basis of model Trainability. 'Trainable model' methodology addresses the issue of the chemical space of ‘in-house’ libraries being considerably wider than that of publicly available data which results in limited applicability of most third-party QSARs for analysis of ‘in-house’ data. The ‘Training engine‘ makes appropriate corrections for systematic deviations produced by the baseline QSAR model based on analysis of similar compounds from the experimental data library. Expansion of this Self-training Library with user-defined experimental data for new compounds leads to instant improvement of prediction accuracy for the respective compound classes. Moreover, addition of 'in-house' data allows adapting the existing model to the particular experimental protocol used in your company and avoiding potential issues related to discrepancies between different experimental methods used for determination of drug interactions with P-gp. | The presented method also forms the basis of model Trainability. 'Trainable model' methodology addresses the issue of the chemical space of ‘in-house’ libraries being considerably wider than that of publicly available data which results in limited applicability of most third-party QSARs for analysis of ‘in-house’ data. The ‘Training engine‘ makes appropriate corrections for systematic deviations produced by the baseline QSAR model based on analysis of similar compounds from the experimental data library. Expansion of this Self-training Library with user-defined experimental data for new compounds leads to instant improvement of prediction accuracy for the respective compound classes. Moreover, addition of 'in-house' data allows adapting the existing model to the particular experimental protocol used in your company and avoiding potential issues related to discrepancies between different experimental methods used for determination of drug interactions with P-gp. | ||
Latest revision as of 10:06, 15 June 2017
Overview
P-glycoprotein (P-gp) is a clinically relevant efflux transporter that extrudes compounds from a large variety of cells. Its function has been associated with the drugs’ absorption, distribution, excretion, CNS effects, multidrug resistance (MDR). P-gp transports a variety of natural compounds and drugs of different therapeutic areas.
Rapid identification of drug candidates that are P-gp substrates and/or inhibitors is possible using P-gp specificity module. Filtering and exclusion of P-gp substrates/inhibitors from huge ‘in-house’ libraries of synthesized compounds or virtual libraries is possible, followed by exclusion of such compounds from further development. P-gp specificity module may serve as an initial screen that could replace screening test based on P-gp ATPase activity measurements and partially replace expensive experiments with P-gp expressing cell monolayers and P-gp knock-out animals.
Training of P-gp specificity models with ‘in-house’ data allows producing reliable predictions of P-gp interaction with compounds synthesized in your company.
Features
- The P-gp Inhibition prediction module uses two models to estimate the probability a compound inhibits P-gp and if so, is it a potent inhibitor?
- Additional knowledge-based model classifies compounds as P-gp inhibitors/non-inhibitors on the basis of relevant structural features and basic physicochemical parameters.
- Statistical algorithms calculate estimate the reliability of every prediction by means of Reliability Index calculation.
- Displays experimental data for the 5 most similar compounds from P-gp DB.
- Training set consisted of >1,500 compounds. Reference data were compiled from more than 800 original publications.
Interface
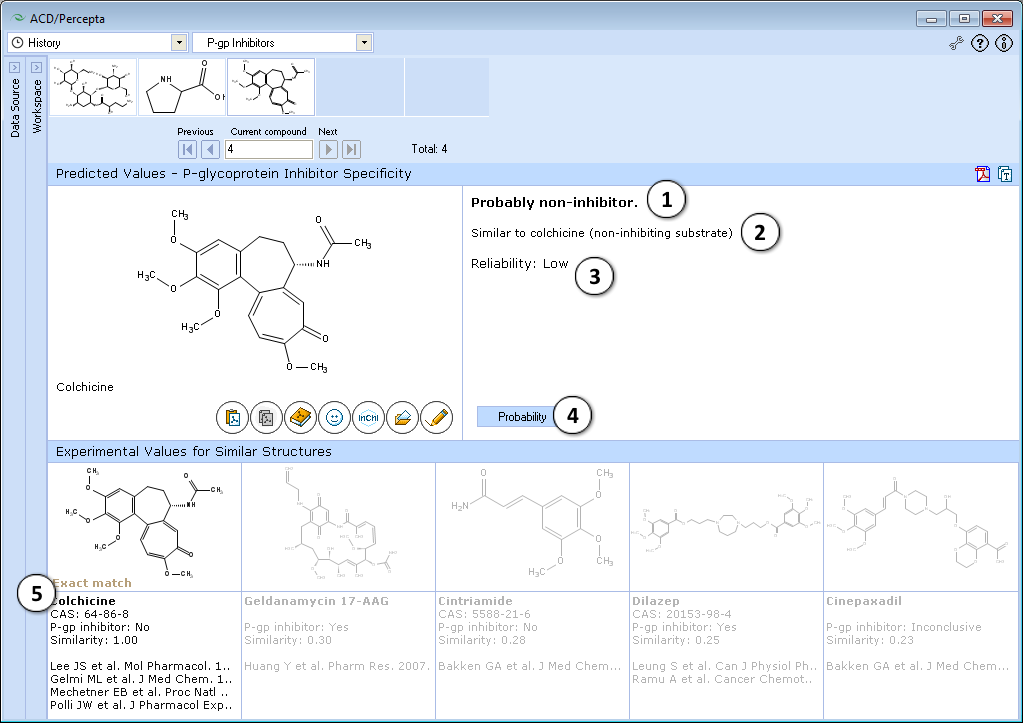
- Classification of compounds as inhibitors or non-inhibitors
- Rule-based reasoning of prediction
- Reliability of prediction (low, medium, high)
- Switch to the probabilistic model
- View 5 most similar structures in the P-gp DB and references
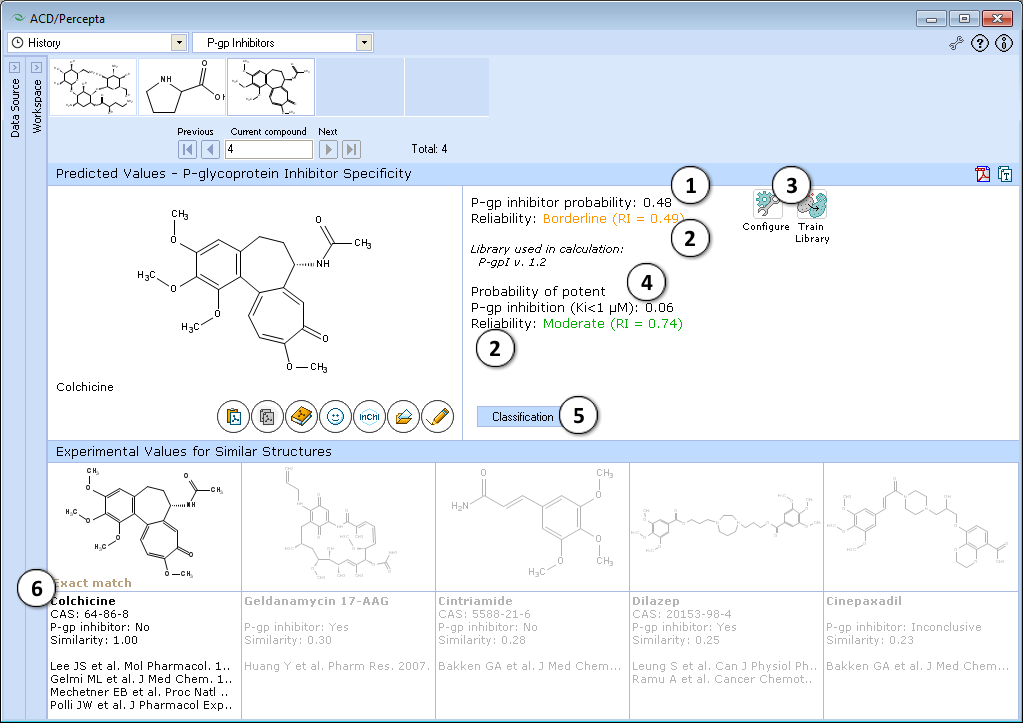
- Probability of the compound being a P-gp inhibitor
- Indication of the prediction reliability (Reliability Index value)
- "Configure" and "Train" buttons provide the means to select the training library for use in calculations and to add new data to that library. The name of the currently selected library is indicated with italic font.
- Probability of the compound being a potent P-gp inhibitor with Ki < 1 uM
- Switch to the knowledge based classification model
- View 5 most similar structures in the P-gp DB and references
Note: Prediction reliability classification according to Reliability Index (RI) values:
- RI < 0.3 – Not Reliable,
- RI in range 0.3-0.5 – Borderline Reliability,
- RI in range 0.5-0.75 – Moderate Reliability,
- RI >= 0.75 – High Reliability
Technical information
Experimental data
There are many in vitro and in vivo tests used in P-gp specificity studies that often produce contradictive results. P-gp specificity model is based on the data collected from scientific literature. The following assays for substrates were considered:
- In vitro polarized transport across P-gp expressing cell monolayers
- In vivo BBB models with P-gp knock-out animals, P-gp mediated drug resistance.
The respective assays for inhibitors were as follows:
- Drug efflux inhibition across/out of P-gp expressing cells
- MDR reversion.
Overall data set for P-gp substrates contains >1000 compounds, for inhibitors >1500 compounds.
Reference database
ACD/Percepta includes a browsable P-gp DB comprised of 2,290 compounds with experimental data related to their interactions with P-gp. Each compound in the DB is classified as a P-gp substrate or non-substrate (inconclusive or contradictive data are marked as Yes/No or No/Yes in the 'Substrate field) and efficiency of P-gp mediated transport is provided for substrates. High efficiency describes compounds that are transported with the rate similar to the best substrates (vinblastine, daunorubicin, paclitaxel). Similarly, drugs comprising the database are classified according to their Inhibitor liability. Potency field denotes effectivity of P-gp inhibition, High potency representing compounds that inhibit P-gp as good as standard inhibitor verapamil or even better.
In the Assays section, the methods that were used in the analysis of P-gp substrate/inhibitor specificity are listed:
- Substrate (in vitro transport assay) – polarized transport of drugs across P-gp expressing cell monolayers or decreased drug accumulation in MDR cells
- Substrate (in vivo BBB models) – increased distribution of drugs to the brain in P-gp deficient (mdr1a/b(-/-)) mice
- P-gp mediated resistance – P-gp overexpressing (MDR) cells demonstrate resistance to the drug
- Drug efflux inhibition – inhibition of drug efflux in P-gp expressing cells.
- MDR reversion – sensitization of P-gp expressing cells to “MDR profile” drugs (taxanes, anthracyclines, vinca alkaloids)
- P-gp ATPase modulation – activation or inhibition of P-gp ATPase. This assay does not differentiate P-gp substrates and inhibitors.
Model features & prediction accuracy
The predictive models of P-gp inhibition were derived using GALAS (Global, Adjusted Locally According to Similarity) modeling methodology (please refer to [1] for more details).
Each GALAS model consists of two parts:
- Global baseline statistical model employing binomial PLS with multiple bootstrapping using a predefined set of fragmental descriptors, that reflects general trends in P-gp inhibitor specificity.
- Similarity-based routine that performs local correction of baseline predictions taking into account the differences between baseline and experimental values for the most similar training set compounds.
GALAS methodology also provides the basis for estimating reliability of predictions by the means of calculated Reliability Index (RI) value that takes into account:
- Similarity of tested compound to the training set molecules (prediction is unreliable if no similar compounds have been found).
- Consistence of experimental values and baseline model prediction for the most similar similar compounds from the training set (discrepant data for similar molecules, i.e. alternating P-gp inhibitors and non-inhibitors lead to lower RI values).
Reliability Index ranges from 0 to 1 (0 corresponds to a completely unreliable, and 1 - a highly reliable prediction) and serves as an indication whether a submitted compound falls within the Model Applicability Domain. Compounds obtaining predictions RI < 0.3 are considered outside of the Applicability Domain of the model.
The presented method also forms the basis of model Trainability. 'Trainable model' methodology addresses the issue of the chemical space of ‘in-house’ libraries being considerably wider than that of publicly available data which results in limited applicability of most third-party QSARs for analysis of ‘in-house’ data. The ‘Training engine‘ makes appropriate corrections for systematic deviations produced by the baseline QSAR model based on analysis of similar compounds from the experimental data library. Expansion of this Self-training Library with user-defined experimental data for new compounds leads to instant improvement of prediction accuracy for the respective compound classes. Moreover, addition of 'in-house' data allows adapting the existing model to the particular experimental protocol used in your company and avoiding potential issues related to discrepancies between different experimental methods used for determination of drug interactions with P-gp.
If the compound is within model Applicability Domain (acceptable Reliability Index) accuracy and sensitivity of classification is close to 90% for both models.